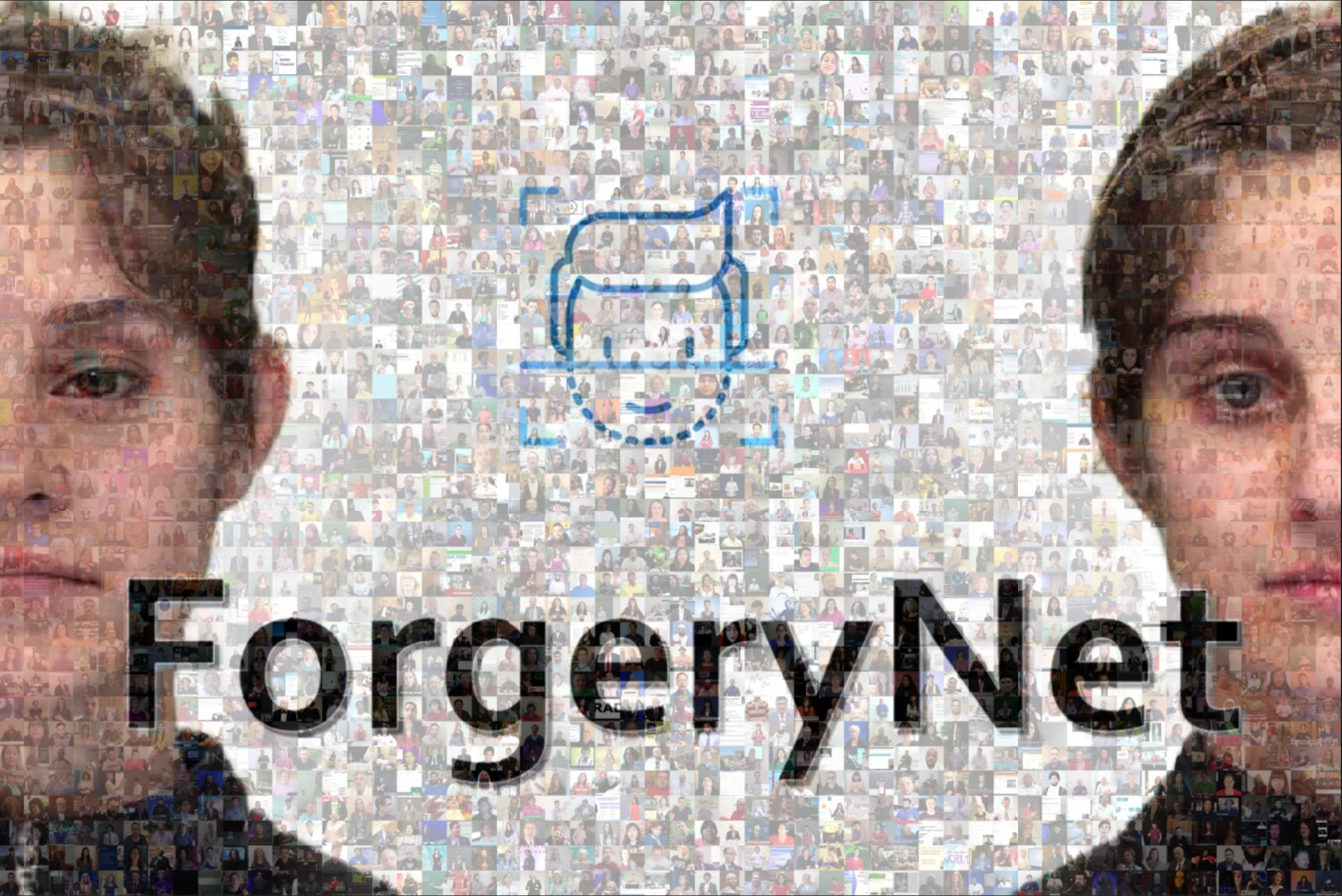
ForgeryNet: A Versatile Benchmark for Comprehensive Forgery Analysis
Yinan He, Bei Gan, Siyu Chen, Yichun Zhou, Guojun Yin, Luchuan Song, Lu Sheng, Jing Shao, Ziwei Liu
Find moreChallenge
Video
Download
ForgeryNet Dataset
ForgerNet dataset contains 2.9 million images and 221,247 videos, 7 image-level approaches and 8 video-level approaches manipulations from all over the world (click "Download * Set" to download). Each video and image is associated with its label (click "Download * List" to download).
MD5:
272d385f3cac5dd6feabc25711c18a41 Training.tar
You should use `cat Training.tar.* >> Training.tar` after downloading the training set from Google Drive.
df320faca2128e022764f8d0e4bc75a1 Validation.tar
e4218faa4b934345977202edfbf89301 public_test_images.tar
92b870009cb03832b2c2795b6a35629f public_test_videos.tar
If you can access Google Drive:
We also provide other way to download:
We construct theForgeryNet dataset, an extremely large face forgery datasetwith unified annotations in image- and video-level dataacross four tasks: 1)Image Forgery Classification, in-cluding two-way (real / fake), three-way (real / fake with identity-replaced forgery approaches / fake with identity-remained forgery approaches), andn-way (real and15respective forgery approaches) classification.2)Spa-tial Forgery Localization, which segments the manipu-lated area of fake images compared to their correspond-ing real images. 3)Video Forgery Classification, whichre-defines the video-level forgery classification with manip-ulated frames in random positions. This task is impor-tant because attackers in real world are free to manipu-late any target frame. and 4)Temporal Forgery Localiza-tion, to localize the temporal segments which are manipu-lated. ForgeryNet is by far the largest publicly availabledeep face forgery dataset in terms of data-scale (2.9 millionimages, 221,247 videos), manipulations (7 image-level ap-proaches, 8 video-level approaches), perturbations (36 in-dependent and more mixed perturbations) and annotations(6.3 million classification labels, 2.9 million manipulated area annotations and 221,247 temporal forgery segment la-bels). We perform extensive benchmarking and studies ofexisting face forensics methods and obtain several valuableobservations. We hope that the scale, quality, and varietyof our ForgeryNet dataset will foster further research andinnovation in the area of face forgery classification, as wellas spatial and temporal forgery localizationetc.